Fostering Data Science
Today, there is a worldwide shortage of data science talent that threatens to derail the Big Data revolution that is transforming business and society. The concept of the citizen data scientist first gained prominence in 2015, when Gartner coined the term, referring to it as “a person who creates or generates models that use advanced diagnostic analytics or predictive and prescriptive capabilities, but whose primary job function is outside the field of statistics and analytics.” Gartner believes today's citizen data scientists can perform the sophisticated analyses that could only be done by data scientists in the past.
As Chief Product Officer at Zerve, a young, stealthy startup that’s rethinking the data science development experience, and an early joiner at DataRobot, Dr. Greg Michaelson achieved the status of “true” Data Scientist over a decade ago, then witnessed the consequences of Big Data in the hands of less qualified individuals over the ensuing years. This breadth of experience has given Michaelson unique insight into the subject of “citizen data scientists.” Interviewer John Krohn looked for unbiased feedback on this controversial topic when he met with Michaelson in February of 2024.
“I think that I once saw a grainy photograph of a citizen data scientist in a national park. I’ve never actually met one.” - Dr. Greg Michaelson
The digital transformation and the rise of Big Data have made the need for data scientists in general, and those who understand business-specific context in particular, greater than ever. Still, industry experts like Michaelson remain skeptical that one individual can successfully embody both roles. “You actually have to have to have a significant amount of knowledge to frame a problem in the first place. The other thing is, you’re gonna need code at some point along the way.”
Michaelson’s skepticism has been echoed by others over the past decade, but the advent of “auto-ML” software to simplify the creation of machine learning code, along with other advanced software tools, are flipping the script and democratizing data. The bar of what can be done by someone without a formal statistics and analytics education has been raised substantially by software vendors who have created business intelligence, data integration, and analytics tools that simplify the data science process so much so that it is literally accessible to everyone.

Humble Beginnings of the Citizen Data Scientist
The concept of the citizen data scientist first gained prominence in 2015, when Gartner coined the term, referring to it as “a person who creates or generates models that use advanced diagnostic analytics or predictive and prescriptive capabilities, but whose primary job function is outside the field of statistics and analytics.” Gartner believes today's citizen data scientists can perform the sophisticated data analysis that could only be done by “pure” data scientists in the past. Utilizing the powerful BI and analytics tools available to them today, citizen data scientists can handle the advanced analytics tasks that were beyond them a few short years ago.
Once the Harvard Business Review dubbed it The Sexiest Job of the 21st Century, the data scientist role became one of the hottest jobs around. As Thomas H. Davenport and D.J. Patil stated in the HBR article, the data scientist’s “sudden appearance on the business scene reflects the fact that companies are now wrestling with information that comes in varieties and volumes never encountered before.” This distinction helped to make the role of the data scientist an attractive one for many non-experts just as software tools were lowering the educational bar.
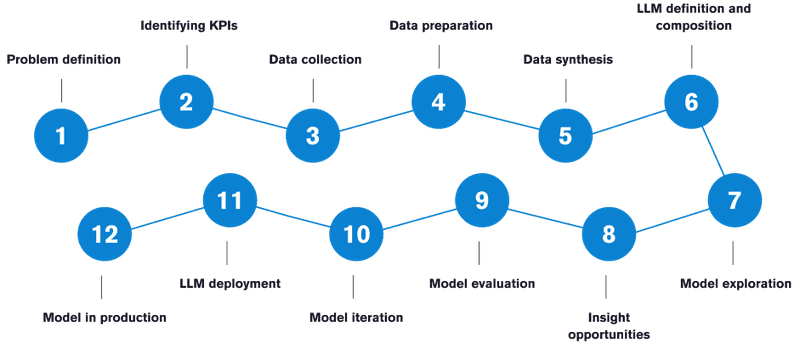
Citizen vs full time data scientist
According to Data Robot, citizen data scientists usually work in departments like sales, marketing, finance, and/or human resources, and possess deep domain knowledge about the department’s unique business challenges. Citizen data scientists perform detailed diagnostic analysis and create machine learning models to supplement work previously done by data scientists, statisticians, and mathematicians. For example, a citizen data scientist might handle the Key Performance Indicators (KPIs) for a specific department while also working together with data scientists on projects that require deeper business expertise. The citizen data scientist will leave the more complex projects that impact the entire organization to the traditionally educated data scientists.

Empowering the citizen data scientist
For TIBCO, the enterprise data company, utilizing citizen data scientists helps businesses get the most value out of their advanced analytics investments while avoiding overspending on expert data scientists. TIBCO believes organizations can empower their citizen data scientists by utilizing a combination of people, processes, and technology. Citizen data scientists might lack formal analytics training but they can still employ a variety of tools that help with:
- Preparing data for analysis to enhance its suitability and relevance.
- Cleansing data to ensure accuracy, completeness, and reliability.
- Creating and refining models for effective analytical representation.
- Recognizing patterns within data to uncover meaningful insights and trends.
- Utilizing deep learning techniques to extract valuable and nuanced data insights.
Citizen data scientists can be Line-of-Business (LOB) staff, business analysts, business intelligence (BI) users, and even members of the IT team. With such a wide net, the citizen data scientist plays an important role within an organization, ensuring data and insights are shared throughout the departments.
Since data is such an important differentiator in business these days, companies can no longer get by without BI and analytics applications. It is imperative to get important information into the hands of the stakeholders, rather than just to the data scientists and analysts. Rather than siloing data, organizations should aim for a holistic data environment throughout the company.
The tools of the citizen data scientist trade
Over the past decade, numerous software vendors have produced extremely powerful solutions that simplify the data integration, business intelligence, data visualization, and analytical modeling process that democratize data. A short list of vendors providing new and improved tools to empower citizen data scientists is reflected in the following market map:

Low-code application platforms (LCAP) provide a visual drag-and-drop environment to simplify the development process and reduce the time-consuming process of hand-coding. Apps can be built quickly and easily on low-code application platforms like Appian, Quickbase, Google App Maker, and Microsoft’s Power Apps. Analytics software providers are also creating data visualization tools that allow data streams to be added, connected, and analyzed via simple drop-and-drag methods.
Data analytics tools like Alteryx are designed specifically for the citizen data scientist and can automate every step of an analytics process, including data prep, data blending, reporting, predictive analytics, artificial intelligence, and machine learning.
“Without big data, you are blind and deaf in the middle of a freeway.” – Geoffrey Moore, management consultant, and theorist.
Data Robot, the Boston-based Data Science company, simplifies the building of predictive analytics solutions and lets users create machine learning models with little technical skills. The user uploads a dataset to Data Robot, then picks a target variable corresponding to the practical business problem they want to solve. The Data Robot platform selects the most appropriate algorithm for the data, and completes the data preparation. Each trained model is ranked according to accuracy, making the results easy for users to interpret.
In search of the citizen data scientist
With the tools, demand, and strategy in place, surely there must be many examples of successful data scientists that can be added to Michaelson’s grainy photo collection? “They end up becoming less and less of a citizen as they get more of that experience,” explains John Krohn. This outlook regards the citizen data scientist as more of “data scientist in training.”
What Howard Dresner, President of Dresner Advisory Services, calls “information democracy” is the idea that data and insights are shared across the organization. “Information democracy ensures every individual has timely, relevant, and actionable insights to successfully carry out the tasks associated with his or her role,” says Dresner. If AI and auto-ML tools are the first key to creating more successful citizen data scientists to meet the demand, then well integrated BI systems are the second.
“Well-implemented embedded BI capabilities support and promote information democracy,” according to TIBCO. This enables more self-service BI, improves report and analysis access, and improves BI collaboration throughout the company. In-context insights and analysis in internal applications make it easier for users to get important insights about their company data.

Self-described citizen data scientist Rishi K of Alteryx described his own journey as a natural evolution where developing better analytical and reporting tools in the investment banking realm led him to share his progress with colleagues and become the de facto Big Data guru, spreading his tools and expertise. “I showed them how these new dashboards allowed them to view and utilize data that, at one point, was difficult to get,” he says. “Once the reluctant individuals recognized this, not only did they embrace the dashboards, but became evangelists for everyone else in finance.”
Rishi’s journey underscores the importance of mentoring as a means to reduce the risks associated with citizen data scientists. Auto-ML tools can generate code quickly, but a trained data scientist is still needed to validate the code to ensure it will not go functionally or technically sideways. For example, unbalanced training data sets and model overfits or underfits will not always be detected by the software tools. Under the wing of an experienced data scientist, the citizen data scientist can also navigate the ethical, legal, and regulatory, risks associated with AI more effectively.
Key takeaways
Skepticism over the existence of citizen data scientists is understandable. Perhaps what is needed is simply a better understanding of the citizen role, and its limitations. Computers have made us all statisticians, artists, and accurate spellers, whether or not we understand the technology that makes these tasks so seamless. So it seems, will be the future of the citizen data scientist.
Under the watchful eye of career AI and Big Data experts, more and more professionals from fields ranging from sales to human resources to finance will have access to both the analytical tools and the raw data needed to perform tasks that could once only be done by a handful of experts. These experts agree that even the best and most user-friendly tools require oversight to ensure the output is as expected, project goals are met, and risks are mitigated.
If you’re an executive, and you drive the hiring, cultivating, expansion, and development of data and its accuracy in the organization, we posit these actionable steps:
- Invest in continuous learning: Support data scientists with resources for skill enhancement, such as funding for relevant courses and conferences. This approach has been successful at companies like Google, where employees are encouraged to pursue further education through initiatives like the Google Learning Center.
- Foster collaboration: Encourage cross-functional teamwork, drawing inspiration from successful models like the one at Spotify, where interdisciplinary squads collaborate seamlessly to drive innovation and problem-solving.
- Recognize achievements: Implement a robust system for acknowledging and rewarding data scientists' contributions, mirroring the effective recognition programs seen at companies like Salesforce, where top performers are celebrated and rewarded publicly.
- Prioritize well-being: Take concrete steps to support work-life balance, following the example set by companies like Microsoft, which offers flexible work arrangements and mental health resources to promote employee well-being.
- Provide access to cutting-edge tools: Ensure data scientists have access to state-of-the-art technologies, as seen in the approach taken by Netflix, where advanced analytics tools empower data scientists to deliver high-quality insights and analysis.
- Encourage risk-taking: Cultivate a culture that values experimentation and learning from failures, akin to the innovative culture at Amazon, where employees are encouraged to take calculated risks in pursuit of groundbreaking solutions.
- Promote diversity: Actively recruit and retain diverse talent to foster creativity and inclusivity, as exemplified by the diversity initiatives at companies like IBM, where diverse teams have been shown to outperform homogeneous ones in problem-solving tasks.
- Offer mentorship: Establish mentorship programs to provide guidance and support for data scientists' career advancement, drawing inspiration from successful mentorship models seen at companies like Facebook, where mentorship is integral to employee development.
- Track progress: Set measurable KPIs to monitor the effectiveness of initiatives in cultivating a data-driven culture and supporting data scientists' well-being, following the data-driven approach embraced by companies like Airbnb, where metrics are used to continuously improve processes and outcomes.